You thought you had everything covered. But when your data annotation vendor gets back with error-filled datasets, you’re left with nothing but frustration. With deadlines to meet, you’re forced to seek a different, and hopefully, more competent data labeling service provider.
Sounds horrifying, but that’s what some of our clients went through before they turned to us.
Like it or not, partnering with a credible vendor can make a difference in your project. But with many vendors to choose from, how do you decide which suits your project needs?
You’ll need to do the due diligence. And this guide will show you:
- Things to look for.
- Red flags to avoid.
- Questions to ask when interviewing vendors.
Let’s start.
Why It’s Worth Being Picky
Data annotation, as you know, is a laborious process that demands precision, collaboration, and consistency. Not only does it require a sizeable team of annotators, but it also calls for coordination amongst project managers, machine learning engineers, domain experts, and annotators.
On paper, you might find certain data annotation vendors attractive, particularly if they’re offering their service at a low price. However, not all vendors are equipped with an internal system that satisfies the project's requirements.
For example, some of our clients initially chose the cheapest vendor, but they ended up with quality issues in their dataset. Likewise, vendors who charge an expensive fee may not guarantee a favorable outcome.
So, it’s better to spend more time assessing vendors before making a choice. Otherwise, you risk costly reworks and project delays. Or worse, deploying a flawed machine learning model that compromises users.
What to Look For in a Data Annotation Company
We know finding the right data labeling company is tough, considering the number of them you’ll find in the market. Still, by vetting the candidates based on several criteria, you can narrow down the list and find a reliable one.
Accuracy & QA Processes
The first thing to look for in any data labeling service provider is a strong quality check mechanism. If the annotated dataset is compromised, so would the resulting machine learning model. Imagine a medical imaging system struggling to identify a malignant tumor due to inconsistent annotations. The outcome? Disastrous.
So, find out how a potential vendor validates their work before contracting them. For example, at CVAT,
- We train our annotators to adhere strictly to the client’s labeling guidelines.
- Then, we assess the annotated datasets with quality assurance techniques like Ground Truth, Consensus, and Honeypots.
- We provide a detailed QA report to our clients and are open to refining the dataset.
Don’t stop at understanding the annotation process, but go a step further by requesting a proof of concept (PoC) from the vendor. In fact, we strongly recommend this move as it gives you stronger confidence in the vendor’s annotation quality. If the vendor can’t produce quality annotations from a small sample, chances are it won’t be able to in the actual project.
Workforce Setup (Outsourcing vs. In-House)
The way a data labeling company recruits, trains, and manages its annotators can affect your ML project. Some data labeling companies don’t have an internal annotation team. Instead, they rely on outsourced labelers, which they don’t have control over. All they do is act as an intermediary, pass on the jobs, and make a profit out of it.
On the other hand, data annotation companies with an in-house labeling team can better adapt to changing project requirements. Such companies also have tighter control over who they hire as annotators, as well as the training that labelers undergo.
At CVAT, we don’t outsource annotation jobs to others. Instead, we implement every annotation job we take and directly communicate the outcomes to our clients. Moreover, we thoroughly vet each annotator we hire. They’re put to tasks with test projects before we onboard them to our global annotation team. A professional team spread across the world is how we can offer 24/7 project execution across time zones.
So, go for a data annotation company that operates with a professional in-house team, particularly if you’re training a complex model. Otherwise, be prepared to deal with noisy datasets, delays, or both.
Security and Compliance
The last thing you need is to suffer a data incident when you trust the vendor to keep your datasets safe. But such a scenario could happen if the vendor you appoint isn’t well-equipped with data security measures. Likewise, partnering with data annotation companies that fail to comply with data privacy laws like GDPR, CCPA, or HIPAA can invite legal troubles.
So, the next time you’re evaluating data labeling vendors, find out how they handle data. At the bare minimum, they need to implement compliant measures to protect datasets from intentional or unintentional breaches. For example, we protect our clients’ data by:
- signing an NDA before commencing the project.
- complying with data privacy laws like GDPR in our workflow.
- applying security measures such as secure cloud integration.
- imposing controlled access on datasets to authorized personnel.
Domain Expertise
Some data annotation projects require domain experts to be part of the annotation workflow. Otherwise, the dataset they deliver might not be precisely labeled. For example, if you’re working on a medical imaging system that trains on medical datasets, you need trained annotators capable of differentiating tumors, fractures, and other anomalies.
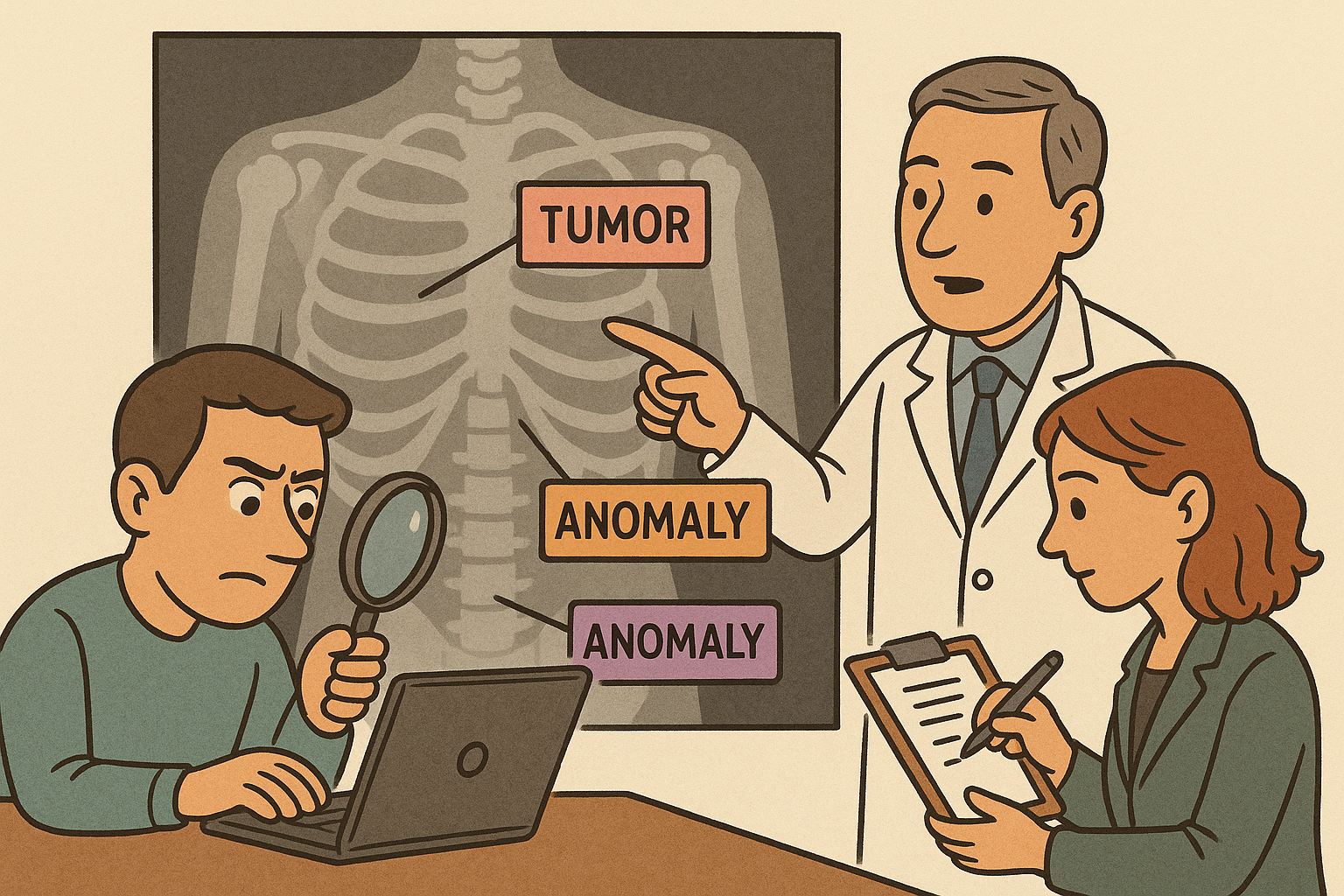
A quick way to check if the vendor has the required expertise is through their portfolio and case studies. If they’ve worked on a similar project in your industry, they are most likely a good fit compared to others. Otherwise, follow what our clients do — assess the vendor through a PoC. Then, decide if they live up to their marketing pitch.
Scalability & Turnaround Time
Most companies innovating with AI/ML models start with a simple prototype, which their vendor has no issue annotating. But as they grow, they need to annotate objects with diverse complexities and types. And that’s where operational limits, if any, start to show.
With changing requirements, some data labeling vendors struggle to cope, resulting in costly delays. Worse, if they fail to adapt to new requirements, you will need to seek a different provider and adapt to a new workflow all over again.
So, how do you spot scalability issues BEFORE you start a project?
One giveaway is vendors who delay starting a project because they lack resources. Also, you might want to reconsider your option if the vendor charges more to prioritize your project.
Andrey Chernov, Head of Labeling at CVAT, explains:
“Make sure your vendor can clearly explain how they run their process and back up any promises they made.”
As a precaution, find out if the vendor can cope with growing annotation workloads as your project scales. On top of that, you can also ask for the typical turnaround time that the vendor can commit to. At CVAT, most projects take 1 month to complete, but we strive to deliver faster.
Pricing
We’ve mentioned that pricing shouldn’t be a deciding factor when choosing a vendor. That said, price can be a useful guide, especially if vendors demonstrate quality in their pilot test.
Another consideration is your budget, which the vendor’s price must fit into. And that’s where transparency comes into play. The vendor you choose should be upfront about the fee they charge, because no one enjoys hidden surprises.
At CVAT, we price a project based on the following models.
{{service-provider-table-1="/blog-banners"}}
We don’t rush into a contract straightaway. Instead, we will work through your project requirements, list the tasks involved, and offer a transparent pricing model. Once we finalize the price, we’ll honor it throughout our engagement. Yes, no rude surprises for our clients. On top of that, we also provide volume discounts to clients as we encourage long-term partnerships.
Tip: To protect your interest, we strongly recommend that you finalize the price with the vendor and lock it with a contract. That’s the practice we do at CVAT to prevent misaligned expectations with our clients.
Red Flags to Avoid
The harsh reality is that not all data labeling service providers are committed to delivering high-quality results. Thankfully, you can call them out by some obvious traits they show.
Lack of process transparency
The vendor should be able to clearly explain their data annotation workflow. From data storage to how they distribute labeling tasks to annotators, a good vendor will take you through the stages — patiently. So, if all you get are vague responses, be wary about engaging that particular vendor.
Unrealistic promises
Ever met vendors that promise 100% annotation accuracy before understanding your project requirements? Well, that’s a major red flag. Any vendor worth collaborating with will take the time to ask questions, ask for representative data, and run a PoC before promising anything.
Communication barrier
If the vendor struggles to provide feedback to your development team, you may want to consider other options. Clear and timely communication, as we know, is pivotal to delivering quality datasets.
Lack of expertise
Some vendors are adept in a specific industry, such as automotive, but unproven in others, like medical and agriculture. If you choose to go ahead, despite knowing the mismatch, you’re risking your project.
In-House vs. Outsourced: Pros & Cons
Amidst frustration, you might consider setting up an in-house data annotation team. But before you do that, consider the pros and cons of doing so.
{{service-provider-table-2="/blog-banners"}}
Having your own in-house labelers naturally provides greater control, but you’ll need to invest in setting up and scaling the team. Not all companies, especially smaller ones, can afford to invest in a team of labelers and annotation tools.
Outsourcing, meanwhile, is more affordable, flexible, and allows you access to highly trained experts. When you outsource, you save resources and time that you can allocate to your core business area.
Of course, we don’t deny the risks of outsourcing, such as data security, compliance, and quality control. However, with careful deliberation, you can find a service provider that addresses your concerns.
For example, our data labeling pipeline is designed to be secure, expert-led, and scalable. Plus, all our feedback goes directly to your project team.
Questions to Ask a Vendor
Ideally, ask clarifying questions before you collaborate with a data labeling service provider. They’ll help you resolve doubts, along with questionable vendors. Below are some questions we thought helpful.
- What industries and types of data have you annotated before (e.g., images, video, text, audio)?
- Can you handle projects with large datasets?
- Are your annotators in-house or crowdsourced?
- What quality control processes do you have in place?
- What is your average annotation accuracy rate?
- What is your average turnaround time for similar projects?
- How do you protect sensitive or proprietary data?
- Do you have your own annotation platform, or do you work with client platforms?
- Do you support domain-specific expertise?
- How is pricing structured (per task, per hour, per dataset)?
- How often will we receive progress updates?
- Do you offer a small paid or unpaid pilot project before full engagement?
Getting Started with a Trusted Provider
Quality annotation is extremely important to ensure that ML models make accurate inferences. But not all data labeling service providers can live up to their promise. We hope you’ve learned how to find one with this guide.
Otherwise, consider partnering with us. CVAT helps companies of all sizes produce accurate, consistent and efficient data annotation. Led by data annotation experts, here is what CVAT has to offer:
- High-quality annotations - We impose strict quality controls to ensure that the annotated datasets meet your requirements.
- Scalable workforce - We vet, hire, and train annotators worldwide to take on annotation projects of all sizes.
- Timeliness - We respect the time we agreed on with our clients and strictly adhere to deadlines.
- Platform expertise - We annotate with the annotation tools we created, putting our team at an advantage against others.
- Seasoned professionals - Our team doesn’t just label data; we know the intricacies of data and communicate directly with our clients.
Brands like OpenCV.ai, Carviz, and Kombai trusted us with their annotation projects. And we hope you will, too.
Want to skip the trial and error? Check out CVAT’s labeling services or get in touch.